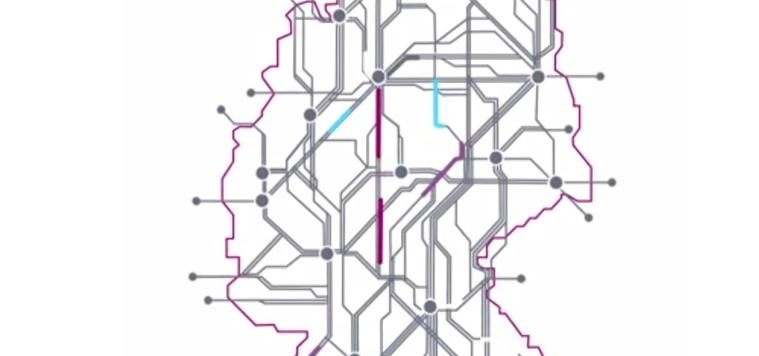
Capacity & Traffic Management System (CTMS)
An intelligent traffic management system is an important building block for the digitalization of network and train operations. In the event of disruptions to operations, a new AI-based "Capacity & Traffic Management System" (CTMS) will optimize train traffic in a matter of seconds.
Project duration
Our partners
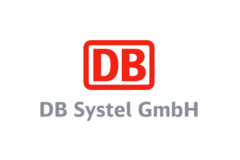
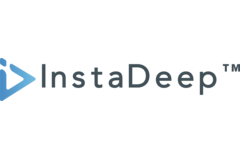
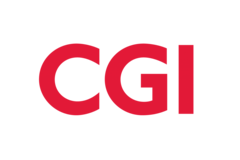
Digitale Schiene Deutschland is developing the Capacity & Traffic Management System (CTMS), an automated planning and control system that uses artificial intelligence (AI) to plan train journeys and construction windows holistically and implement them operationally. If there are disruptions to operations, the CTMS adjusts the plans made in near real time and implements them in conjunction with other innovative components of the digital rail system. It continuously obtains all relevant information from vehicles and infrastructure and incorporates this into its detailed schedules. The plans are created using various optimization approaches, the most advanced and powerful of which is deep reinforcement learning, a branch of AI.
The German rail network today: 33,000 kilometers, 40,000 train journeys, 3 million passengers a day and 350 million tons of freight a year. And the demand for rail capacity continues to grow. In order to achieve the agreed climate targets, even more traffic must be transferred from road to rail and significantly more trains must be added to the existing rail network. However, in dense traffic, disruptions occur time and again at various points. Today's planning and scheduling still largely react to this manually. This will no longer be possible in the even denser traffic of the future: decisions will have to be made quickly, proactively and automatically.
The central building block for this is being developed at Digitale Schiene Deutschland (DSD): the Capacity & Traffic Management System (CTMS), which has the capacity of DB InfraGO AG's rail network as its central optimization object. Its aim is to optimally distribute network capacity on the basis of user requirements. This applies to moving and parked trains as well as network restrictions (e.g. for construction sites). To this end, the CTMS makes microscopically detailed schedules for vehicles and infrastructure and implements these schedules operationally. It makes plans for both the immediate and the distant future. This blurs the current boundaries of planning and scheduling.
The current CTMS prototype plans up to 400 train journeys over several operating hours on medium-sized network corridors of around a thousand kilometers of track. Planning is based on (imitated) requests from railroad undertakings (RUs) for train journeys with a given start, destination and scheduled stops as well as track preferences at stations. The optimization goals are the shortest possible routes and compliance with customer time requirements. The schedules created are precise to the nearest meter and second and contain the necessary requests for points setting and the issuing of run clearances to DSD's digital train protection system (Advanced Protection System, APS). The prototype reacts to unplanned line disruptions by independently rescheduling all directly and indirectly affected train movements, which requires far less than one minute of computing time for 30-35 trains.
Für die Optimierung kommt im Wesentlichen „Deep Reinforcement Learning“ (bestärkendes Lernen) – ein Teilgebiet der Künstlichen Intelligenz (KI) – zum Einsatz. Diese KI sowie andere Optimierungsansätze sowohl aus dem Bereich des Operations Research (OR) als auch hybride KI/OR-Ansätze, werden mit Unterstützung industrieller und akademischer Partner ebenfalls bei der Digitalen Schiene Deutschland entwickelt.
The first operational use of the CTMS is planned for the Digital Node Stuttgart (DKS). By 2030 at the latest, the CTMS will initially control parts of the S-Bahn traffic there via ATO GoA 2 (Automated Train Operation, Grade of Automation 2) on the basis of a digital signaling technology (DSTW) with ETCS Level 2. The control area of the CTMS will then be successively extended to the entire DKS, including regional, long-distance and freight traffic. The interfaces required for the CTMS are to be implemented as early as the 2025/2026 timetable change. A "translator" will use these to supply the ATO with (initially static) journey information until the CTMS goes into operation.
This project will be implemented in Berlin, Frankfurt, Paris and other locations.
- Increased capacity and operational quality through
- Optimization of timetable changes in (near) real time
- Automated execution of timetable changes in (near) real time on infrastructure and vehicles
- Integration of timetable and construction planning
- Increased robustness and stability through scheduling with a network-wide focus - resulting in fewer secondary delays
In cooperation with TU Darmstadt, a software module is being developed in this project to connect CTMS to an external railroad simulation for the first time. The software module takes over the timely control of the infrastructure and delivers data from the driving operation back to CTMS in real time.
By connecting to an external railroad simulation, selected functionalities of CTMS can be observed and verified independently of the previous virtual test environment. The simulation of external disruptive influences provides a more realistic representation of railroad operations. The testing provides valuable insights into the stability and quality of the timetable planners calculated by CTMS and for the structured further development of CTMS.
Project data:
Start: October 2023
End: December 2024
Project partners:
- Eisenbahnbetriebsfeld Darmstadt
- TU Darmstadt
In the REINFORCERAIL project, two methods are being researched and tested as examples to further improve automatic dispatching in CTMS. In addition to the two major infrastructure operators SNCF and DB InfraGO AG, a French and a German university are also involved in this bi-national project.
The first method aims to further accelerate optimization in CTMS by estimating the possible spatial and temporal extent of a disruption of operations using AI methods before optimization.
In the second method, AI agents are combined with operations research methods, allowing the quality of solutions to be controlled and thereby promoting the confidence required for the implementation of a highly automated application.
Project data:
Start: 2023
End: 2027
Project partner:
- SNCF
- Université Gustave Eiffel
- TU Dresden
The EU-funded AI4REALNET (AI for REAL-world NETwork operation) project aims to advance the development of trustworthy AI solutions for critical infrastructure (rail, power grid and air traffic). The focus is on the core results:
- Innovation und KI-Entwicklung: Im Rahmen von AI4REALNET werden Entscheidungsfindungsmethoden der nächsten Generation entwickelt, die Supervised Learning (SL) und Reinforcement Learning (RL) miteinander verbinden. Die Entwicklung von KI-Algorithmen wird durch realistische digitale Simulationsmodelle unterstützt.
- trustworthy AI: AI4REALNET is developing concepts that make AI systems transparent and explainable so that they can be accepted by human operators where necessary.
- human-AI collaboration: In addition to highly automated AI solutions, AI4REALNET also focuses on joint learning between AI and human operators. In this way, automated decision-making is better and successively implemented in operational reality.
The principles of AI4REALNET are already being incorporated into CTMS development at Digital Rail for Germany. With the results of the project, the CTMS can further expand its capabilities to generate not only theoretically good, but also operationally sensible timetable planners and dispatching decisions that are accepted by experts.
Project data:
Start: 2023
End: 2027
Project partners:
- Institute for Systems and Computer Engineering
- Technology and Science
- Institute for Technological Research SystemX
- Fraunhofer Institute for Energy Economics and Energy System Technology
- University of Kassel
- Polytechnic University of Milan
- University of Amsterdam
- Delft University of Technology
- Zurich University of Applied Sciences
- University of Applied Sciences and Arts Northwestern Switzerland
- Linköping University
- EnliteAI GmbH
- Reseau de Transport d’Electricite
- TenneT TSO B.V.
- Swiss Federal Railways
- NAV Portugal, EU
Videos
Capacity & Traffic Management System (CTMS): Trains are being re-scheduled after a disruption
This video illustrates how the CTMS prototype adjusts the schedules of nearly 40 trains during (simulated) live operations. This adjustment is done in a holistic way, even changing schedules of trains that are not directly affected, in order to minimize overall delay with respect to the original schedule. The underlying optimization algorithms are based on Artificial Intelligence (AI).
Explainer Capacity and Traffic Management System
In future, an AI-based capacity and traffic management system will plan and control tens of thousands of train journeys per day in real time in a highly automated manner. The AI method at the heart of the system is called "Deep Reinforcement Learning". This video explains what this means and how this method can revolutionize the planning and scheduling of trains in the future digitalized rail system.
Presentation - Automatic rail and operations control with CTMS
Dr. Michael Küpper, Capacity & Traffic Management Ambassador at Digitale Schiene Deutschland, gave a presentation on this topic at the 28th Safety Technology Conference at TU Dresden on 28-29 September 2023.
Publications
-
On the transport benefits of Digital Rail Germany | February 2024 (only in German)
A study conducted by VIA-Con/quattron on behalf of Digital Rail Germany/DB InfraGo AG reveals the potential of digitalization for increasing rail capacity. In the best-case scenario, the use of digital technologies could lead to around 15% additional marketable train paths and a significant increase in operational quality.
Source: Der Eisenbahningenieur
-
Building the Traffic Management of the Future with Artificial Intelligence | January, February 2024
An intelligent Traffic Management System is a fundamental centerpiece of future digitalized railway network operations. When thousands of trains are on the move in dense traffic, rapid decisions must be made to adjust the operations in the event of disruptions. New approaches are needed in order to be able to respond quickly and effectively to disturbances in future railway operations. Combining Artificial Intelligence (AI) and methods from Operations Research can help address this challenge. This approach was tested on a specific application.
Source: Signal+Draht
-
The Capacity & Traffic Management System for Digital Rail | October 2023 (only in German)
A consistent, intelligent traffic management system for planning and controlling rail operations will form the basis of future automated driving and network operations. This article describes the intended scope of a new, digital and AI-based "Capacity & Traffic Management System" (CTMS), its functionality and the current status of development by Digitale Schiene Deutschland.
Source: Der Eisenbahningenieur