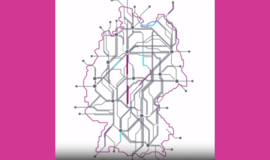
Automatic Planning and Controlling of Rail Operations: From Vision to Prototype
One of the largest potentials for increasing capacity and quality in the rail system – without building new tracks – lies in the automatic, overall optimization of rail traffic. The advancing digitalization and the associated availability of live data as well as new technologies and AI methods such as Deep Reinforcement Learning offer the opportunity to leverage this potential. In the target state, real-time control of all rail traffic will be possible. Traffic demand and resources can then be optimally matched at any time. With the Capacity & Traffic Management System (CTMS), Digitale Schiene Deutschland is developing an important building block for such a digital transport system. The current CTMS prototype already demonstrates how this works, in a small-scale example.
In a future, fully digitalized world of passenger and freight transport, all relevant information would be available in near real-time:
1. Transportation needs
2. Availability of vehicles
3. Availability of infrastructures
Scenarios like this one would become possible: Based on the current number of spectators in the stadium, it is already known at half-time that ten buses and five S-Bahn trains more than planned will be needed to pick up passengers after the sports event. The system (or rather a network of systems) plans the required trips and assigns vehicles correspondingly. Further, the system has the information that one of the S-Bahn railcars will need to undergo maintenance after its newly planned route of 42 km, as the diagnostic sensors on board the railcar permit its brake system a maximum of 50 km to run. The system therefore directs the train to the nearest repair workshop after its last passenger stop and orders an additional maintenance team for the following morning shift.
This scenario is still a vision of the future. However, a central component for the realization of this example is being developed at Digitale Schiene Deutschland (DSD): the Capacity & Traffic Management System (CTMS), which focuses on optimizing the capacity utilization of the DB InfraGO rail network. It aims to optimally distribute the network’s capacity based on its users’ requirements. This applies to moving and parked trains as well as network restrictions (e.g. for construction). For this purpose, the CTMS creates microscopically detailed schedules for vehicles and infrastructure, and it implements these schedules in operation. It makes plans for both the immediate and the distant future. This dissolves the current borders between planning and scheduling.
The current CTMS prototype plans up to 400 train journeys over several operating hours on medium-sized network corridors of around a thousand route kilometers. Planning is based on (imitated) requests from Railroad Undertakings (RU) for train journeys, each with a given start, destination, traffic stops, and track preferences at stops. Optimization targets are shortest possible routes and compliance with customer/RU time requirements. The schedules created are precise down to the level of meters and seconds. They also contain all necessary requests for the switching of points and the issuance of movement authorities that are to be processed by DSD's Advanced Protection System (APS).
Today, the plans are executed in a microscopic emulation. The next step will be to use the CTMS to control a model train system. In the current, emulated live operation, the CTMS prototype can be informed of disruptions. The prototype reacts to disruptions by appropriately rescheduling all running (and still-to-run) trains. The CTMS does this by looking at the entirety of train traffic, regardless of whether a train is directly or indirectly affected by the disruption (see illustration). The optimization target in the event of a disruption is to minimize the overall delay of all trains with respect to the previous plan.
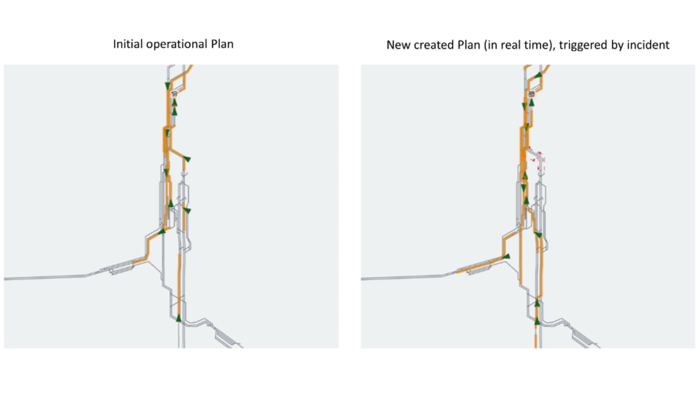
The optimization is mainly based on Deep Reinforcement Learning – a method of Artificial Iintelligence (AI). This type of AI as well as other optimization approaches are, too, being developed at Digitale Schiene Deutschland with the support of industrial and academic partners. Partners include InstaDeep Ltd with headquarters in London and locations worldwide. This coming February, the journal "Signal & Draht" will publish an article by Digitale Schiene Deutschland titled "Artificial Intelligence for the traffic management system of the future". We will report this on our online channels.
The first operational use of the CTMS is planned for the Stuttgart Digital Node (DKS). BBy 2030, the CTMS will initially control parts of the S-Bahn traffic there via ATO GoA 2 (Automated Train Operation, Grade of Automation 2) based on a Digital interlocking (DSTW) with ETCS Level 2. The control area of the CTMS will then successively expand to the entire DKS, including regional, long-distance and freight traffic. The interfaces required by the CTMS are to be implemented as early as the timetable change of 2025/26. A "translator" will then use these interfaces to supply the ATO with (so far static) journey data until the CTMS has gone live.
A recent study by VIA-Con/quattron, on behalf of DB InfraGO AG, of the expected benefits of Digitale Schiene Deutschland has examined the specific impact of the CTMS for the first time. Another Digitale Schiene Deutschland article reporting exclusively on the results of that study will appear in the February issue of the journal "Der Eisenbahningenieur". We will report on this in near future.
Further information: