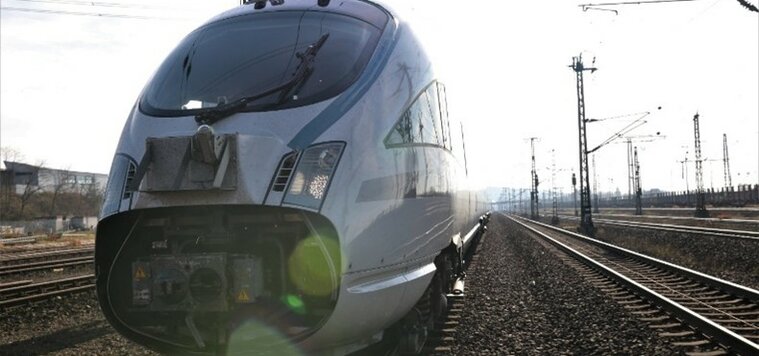
KI-MeZIS
In order to increase the quality of train operations and support them with smart maintenance functions, Digitale Schiene Deutschland participated in the funding project “AI methods in condition monitoring and needs-based maintenance of rail vehicle structures/KI-Methoden in der Zustandsüberwachung und bedarfsangepassten Instandhaltung von Schienenfahrzeugstrukturen (KI-MeZIS)”, which was launched at the end of 2021. The aim of the project was to tap into the potential of artificial intelligence (AI) for monitoring train movements. To this end, sensors for impact and rollover detection were attached to the front and chassis of various test vehicles.
Project duration
Our partners
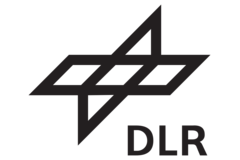
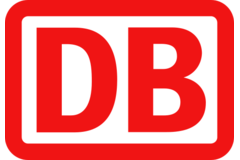
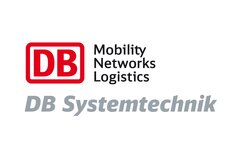
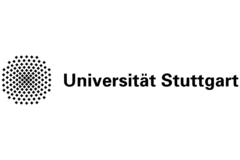
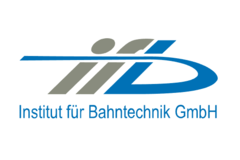
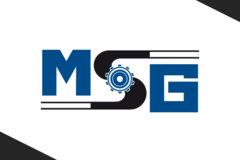
Promoters
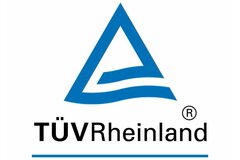
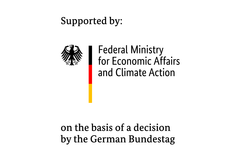
The introduction of fully automated driving is one of the most important fields of action for more efficient and reliable rail operations. Through the work of Digitale Schiene Deutschland and its partners, trains are being equipped with modules for Automatic Train Operation (ATO) or state-of-the-art sensor technology for environment recognition and localization, among other things. These technologies have already been tested in two different pilot projects (Digital S-Bahn Hamburg and Sensors4Rail). In order to enable fully automated, driverless travel in heavy rail systems, further innovative technological components must be incorporated into the future rail system. One of these components is impact detection. It ensures that collisions and impacts at the front of the train as well as rollovers are correctly classified and appropriate reactions for automated driving are derived.
In order to increase the quality of train operations and support them with smart maintenance functions, Digitale Schiene Deutschland was participating in the funding project “AI methods in condition monitoring and needs-based maintenance of rail vehicle structures/KI-Methoden in der Zustandsüberwachung und bedarfsangepassten Instandhaltung von Schienenfahrzeugstrukturen (KI-MeZIS)”, which was launched at the end of 2021. The aim of the project was to tap into the potential of artificial intelligence (AI) for monitoring train movements. To this end, sensors for impact and rollover detection were attached to the front and chassis of various test vehicles. One prominent test vehicle is the so-called "advanced TrainLab" (aTL) - Deutsche Bahn's moving laboratory - a class 605 ICE multiple unit that serves as a test vehicle for new technologies (see illustration).
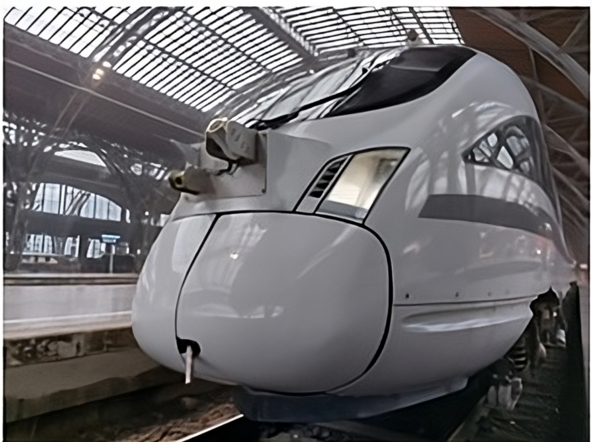
With the help of an AI, the data from the sensors on the front of the train and on the chassis is evaluated and interpreted. This allows collisions to be detected and conclusions to be drawn about the condition of the vehicle. The AI decides whether a train journey must be terminated immediately after impacts, rollovers, damage or collisions or whether it can continue to the next station or a nearby workshop. The recorded sensor data is also used for strategic maintenance and the needs-based design of future vehicles. In addition to the advanced TrainLab (aTL), freight cars are also used to carry out rollover tests.
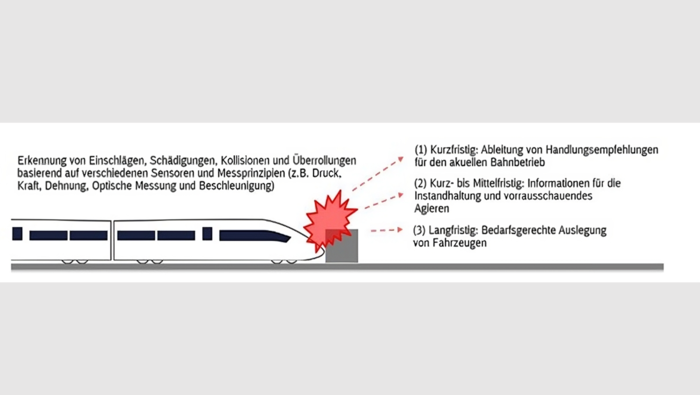
The project was funded as part of a funding call of the Federal Ministry for Economic Affairs and Climate Actions. In addition to DB InfraGO AG, other partners included the Data Intelligence Center (DB AG's AI Factory), the German Aerospace Center (DLS), Industrial Analytics GmbH and the University of Stuttgart. DB InfraGO AG was supported operationally in the project by DB Systemtechnik GmbH, Institut für Bahntechnik GmbH (IFB) and Maschinenbau und Service GmbH (MSG). Other associated partners were J.M. Voith SE & CO. KG, Group Division Turbo, Stadler GmbH and train outfitter Dellner GmbH.
In order to record data from regular rail operations, a sensor system was installed on the train. Critical structures that are particularly affected in the event of an impact or load case were first identified.
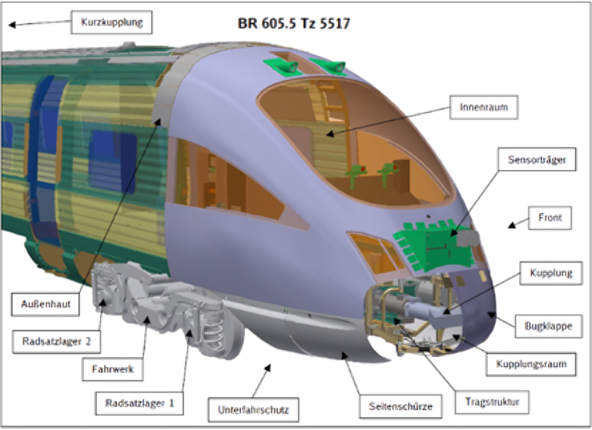
Suitable sensors were then selected. These were designed in such a way that they could also register strong events without overloading. In other words, without the amplitudes being outside the relevant scale range. A total of 43 sensors were installed, whose signals were recorded using a DEWETRON data recorder.
The following sensors were installed:
12 accelerometers
12 extensometers
2 rotary encoders
14 pressure sensors
1 camera
2 temperature sensors
In order to develop an AI-based impact and damage detection system, a large amount of training data was required. The data was obtained from test drives with the advanced TrainLab, rollover tests with a freight car and from laboratory tests with selected components from the front structure of a BR601 ICE and supplemented by simulations. On the one hand, impact and drop tests were carried out with individual vehicle components. On the other hand, the University of Stuttgart (IMA) has created FE models with which crash simulations are carried out. This was used to evaluate how the material and structure react to external forces. The component tests were used to validate the simulations. The test drives with the advanced TrainLab (aTL) were used to generate data that reflects real-life operation. However, actual impacts could not be realized with the aTL. For this reason, impact tests were carried out in the laboratory.
Regular collision events usually occur in the front end area. The nose flaps are particularly affected by collision events. Among other things, impact tests are carried out on them in order to obtain defined acceleration signals for AI-based impact and damage detection. In these tests, both the impact velocities and the test objects were varied. Drop and impact pendulum tests were also carried out. Various materials can be attached to the front of the pendulum.
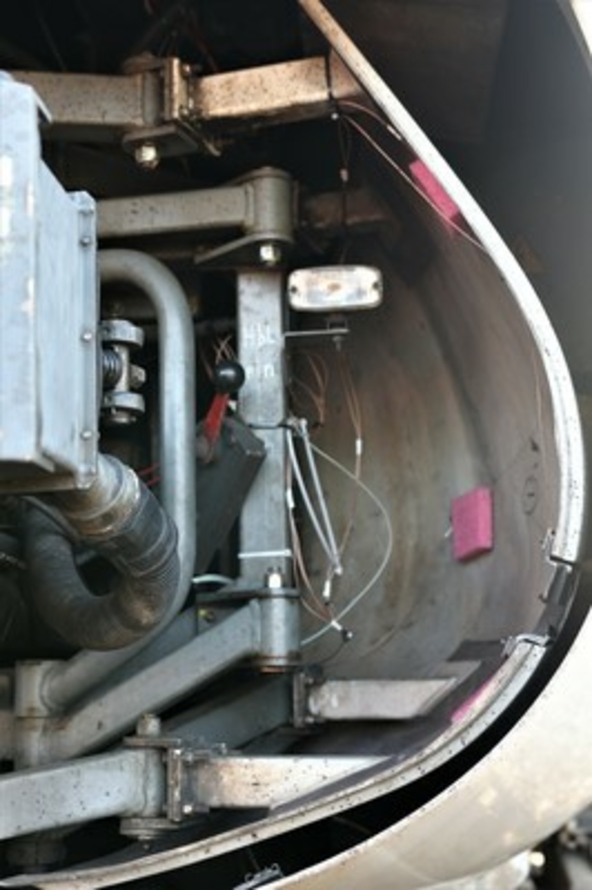
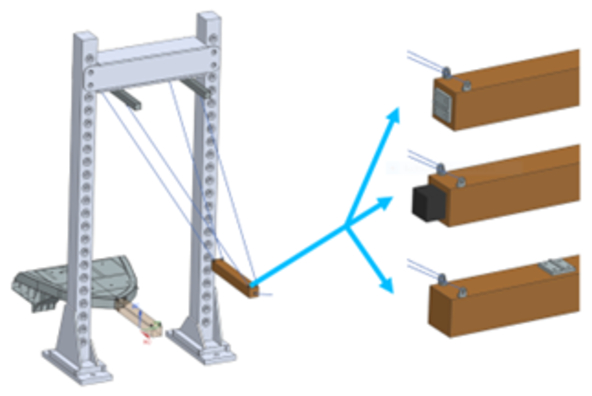
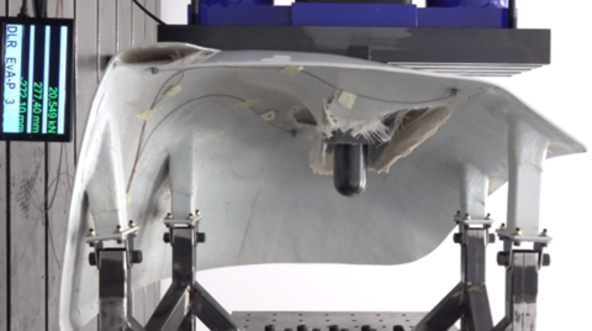
Simulations
The structural behavior of the sensor-monitored components of the aTL in the event of a collision was analyzed using extensive crash and impact simulations. They were based on the so-called "finite element method". Models of the components were subjected to a large number of selected collision scenarios and load conditions. These were derived from realistic crash and impact events in rail traffic. These include, for example, collisions with fallen trees, vehicles, animals and other objects. In the crash and impact simulations, the collision objects were modeled in the form of simplified, representative representations and with corresponding material properties.
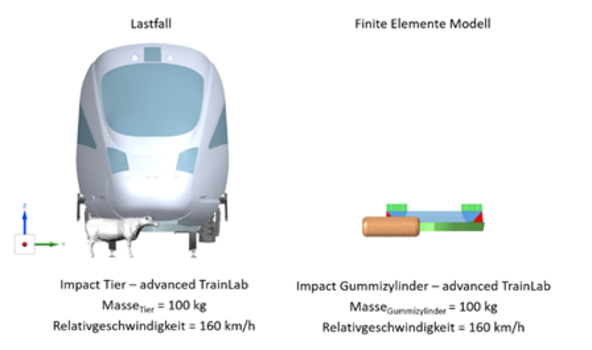
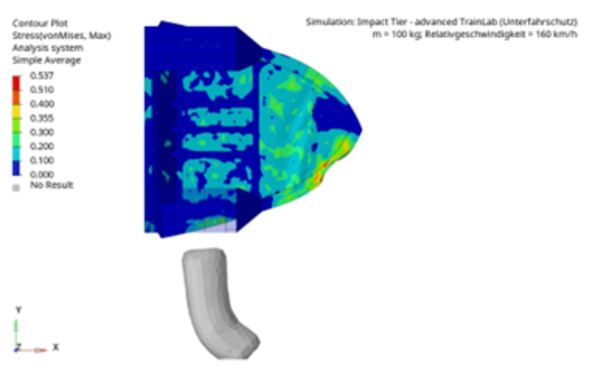
Component tests and simulations with a freight car
A freight car (4-axle flat wagon with side flaps) was used to test rollover events at higher speeds. Accelerometers and rotary encoders were mounted on the truck for this purpose. The sensors are identical to those installed in the aTL. The figure shows the sensor concept on freight cars.
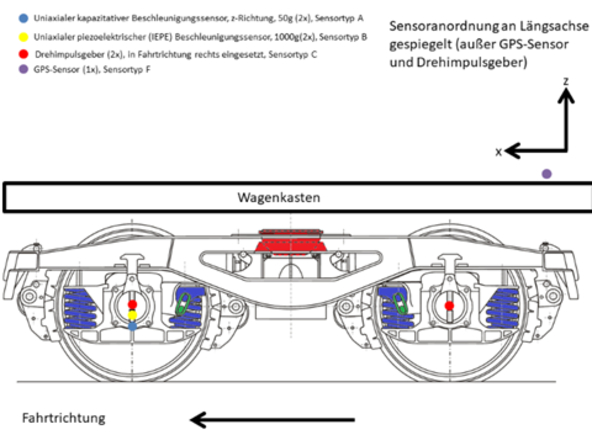
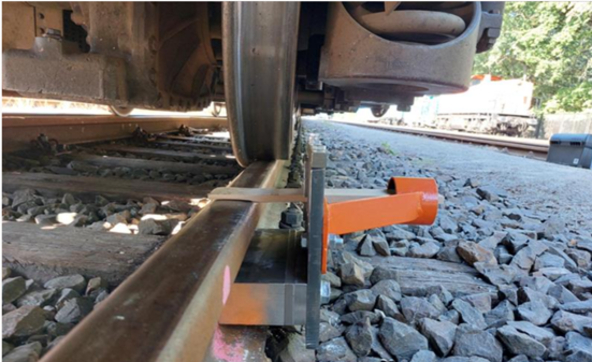
Various objects were driven over at different speeds, such as wood, roof tiles, overhead contact wire, pieces of concrete, animal bones, bicycles and shopping carts.
AI prototype and damage library
In a further work package, the data obtained was evaluated using AI methods. Based on the initial data, a first prototype impact and rollover detection system was implemented. Both threshold-based methods and neural networks were used for this.
The prototype AI methods were being continuously developed and trained with new data. In addition, an "edge device" was installed to enable initial evaluations on the train. The recorded data was then integrated into an "IA dashboard" to ensure the best possible visualization. The dashboard was cloud-based to enable access for all project partners.
In order to be able to provide meaningful recommendations for action on sensor data patterns, different parameters had to be stored in the detection algorithms and their databases. Furthermore, a damage library had to be set up. The damage library was being filled with information based on component tests, train driver surveys and simulation results. An interface design in the form of mock-ups was also created for the damage library, which should enable maintenance engineers and employees to transfer damage directly to the damage library in the future.
The daily mileage of an ICE is up to 1,800 kilometers. During the journeys, the safety-related components and the outer cladding of the train are exposed to various loads. These include, for example, aerodynamic loads (during fast tunnel journeys), recurring tensile and compressive loads (braking, acceleration, coupling processes) or weather-related influences. The components required for this are designed to withstand these loads and guarantee safe rail operations.
To avoid oversizing the components, another research question in this context was: What loads act on the running gear and on the vehicle structure at the front of the train? The aim was to gain insights into the optimal design of the vehicle structure in order to achieve weight optimization. In addition to the detection of impact events, the sensors at the advanced TrainLab (aTL) are used to obtain real measured values. This data is used to develop simulation models that use AI methods to map the operating behavior of these structures more realistically. These can be made available to industry as recommendations in the future.