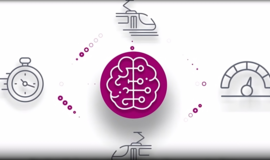
Building the Traffic Management of the Future with Artificial Intelligence
Over 40,000 regional, long-distance and freight train journeys occur daily on the German rail network. When thousands of trains are on the move in dense traffic, rapid decisions must be made to adjust the operations in the event of disruptions. New approaches are needed in order to be able to respond quickly and effectively to disturbances in future railway operations. Combining Artificial Intelligence (AI) and methods from Operations Research can help address this challenge.
An intelligent Traffic Management System (Capacity & Traffic Management System – CTMS) is a fundamental centerpiece of future digitalized railway network operations. A highly automated CTMS will be able to detect disturbances reliably and in advance and create and adapt timetables in real-time throughout the German rail network. Conventional optimization approaches in timetable creation and adaption suffer from an exponential increase of solution time for problems on large networks and/or high numbers of trains, making their application in rail operations impossible in more extensive networks. Combining methods of Operation Research with AI may be a way to tackle this so-called “curse of dimensionality”. The CTMS team of Digitale Schiene Deutschland tested this hypothesis in a realistic dispatching use case on a section of the German railway network centered around the central station of Magdeburg. The results of this study are presented in the journal SIGNAL+DRAHT in detail.
Method and test scenarios
The CTMS team chose infrastructure disruptions in the network mentioned above as a test scenario. In running operations, a current schedule had to be recalculated due to a section of route that was suddenly impassable, and trains had to be rerouted accordingly. The aim was to minimize the overall delay across all running and scheduled trains. The individual delays result from the discrepancy between the new arrival times at the train stations and the time planned in the original timetable. These values are added up across all stops and trains. The more trains, the greater the overall delay. The problem described is referred to in the literature as the Timetable Rescheduling (TTR) problem and has so far been treated using methods from Operation Research (OR). In order to overcome the fact that in OR approaches the computing time for an optimal solution typically grows exponentially with the number of trains, a novel combination of these methods with Deep Reinforcement Learning (DRL) was explored. DRL is an AI method in which AI models learn through experience to solve complex decision-making problems. This method was applied to seven test scenarios, each comprising two hours of operating time and between 20 and 35 trains. The scenarios were generated with a traffic density comparable to the actual traffic density in current operations, especially in the train stations.
Results
In six out of seven scenarios, the results with the new approach were significantly better than with the traditional optimization methods. The computing time of the AI approach for scenarios with 20, 25, 30 and 35 trains was relatively constant at around 10-20 seconds. Importantly, it was not significantly greater for the highest number of 35 trains than for smaller numbers of trains. The tests have shown that the chosen Deep Reinforcement Learning approach has the potential to solve TTR problems in a realistic setting with high quality and that it has favorable scaling properties for larger networks with higher number of trains. This is particularly important for an automated Traffic Management System that has to react flexibly and quickly to disruptions in more extensive network sections. Therefore, further development of such approaches seems very promising.
Further details to this new approach and test scenarios can be found here:
SIGNAL+DRAHT (01+02/2024) „Künstliche Intelligenz für das Verkehrsmanagement der Zukunft“