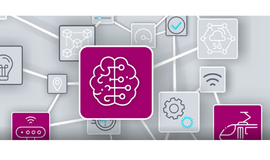
Digitale Schiene Deutschland develops an AI-based capacity and traffic management system using the "Deep Reinforcement Learning" method
In future, an AI-based capacity and traffic management system will plan and control tens of thousands of train runs daily in a highly automated manner in real time. The AI method at the core of the system is called "Deep Reinforcement Learning": Following the model of human learning, AI models are trained through success and failure in a realistic simulation of railway operations.
Fast, AI-based decision-making improves train path scheduling, increases capacity on the rail network, and reduces delays. Experts in artificial intelligence (AI) from DB Netz AG and the AI technology company InstaDeep have been working together on this future topic since 2019 as part of the sector initiative Digitale Schiene Deutschland. In close and intensive teamwork, the foundations were laid for AI in the planning and control of rail transport. Now the cooperation between DB Netz and InstaDeep has been underpinned with a long-term partnership that provides for cooperation for up to seven years. The aim of the cooperation is to develop the optimisation core of the Capacity and Traffic Management System (CTMS). This component of the CTMS makes second-by-second schedules for train movements and infrastructure control possible.
When thousands of trains are driving in dense traffic, quick decisions on how to adjust operations need to be made as disruptions happen. Currently, these adjustments are done by hand or are only supported by IT to some degree with a local focus. This won't be viable in the future due to the increased traffic density. A flexible, fast and effective response to disruptions in dense traffic requires new approaches. Here, an AI-based CTMS can be a promising solution for the future.
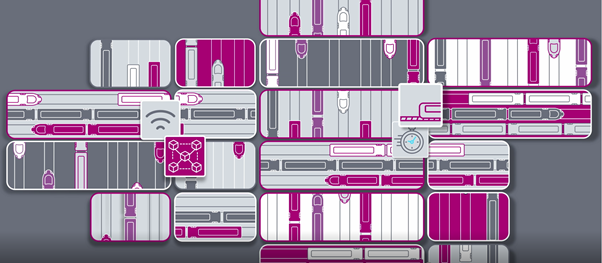
“Deep Reinforcement Learning” was established as the AI method at the core of CTMS: mimicking human learning by experience, AI models learn by “trial-and-error” in a realistic simulated railway environment. In the simulated reality, the AI learns within a representation of the real railway network. The range of experiences during the training extends from simple situations of rail traffic to data from real-life situations and a huge variety of different artificial scenarios. In this way, the AI is trained to be able to react on the tasks and disruption scenarios familiar from today's operations and furthermore with unknown future situations.
What Deep Reinforcement Learning is and how it revolutionizes the scheduling and dispatching in the digital railway system of the future, you can see in the following video:
An initial prototype of the CTMS Optimiser that planes and controls simulated railway operations at a microscopic level was developed since 2019. This has demonstrated crucial properties of such an AI-powered system: scalability, flexibility, and generalization (as described in detail here).
Within the new deal the cooperation between the companies plans to extend the simulation environment and the existing prototypes functionally to cover all must-have functions of the CTMS and its main use cases. The AI framework and methods will be further refined towards AI models that demonstrate the scaling, generalization, and performance properties required for the target system of a fully automated railway system. The ultimate goal is the beginning of a german wide deployment of a production-grade, scalable, and functional AI-powered Optimiser for its Capacity & Traffic Management System. This system will automatically schedule and reschedule trains and infrastructure restrictions, using information from further future technologies and applications, e.g. sensor-based perception systems and a train centric Advanced Protection System that are also developed within the sector initiative Digitale Schiene Deutschland. Via additional safety systems on-board and trackside, the future CTMS aims to optimize and control the movement of up to 40,000 trains a day as well as the integration of maintenance and construction work on the network into the planning process. This will help to achieve our main goals: higher capacity and optimised utilisation of the rail network with more trains and an attractive rail transport with higher quality.