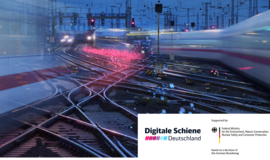
Artificial Intelligence as a game changer for Capacity and Traffic Management in the future railway system
In the context of the sector initiative Digital Rail for Germany (DSD), Deutsche Bahn and its partner firm InstaDeep have developed initial prototypes of a planning and operations control system for railway infrastructure based on Artificial Intelligence (AI). Between November 2020 and December 2021, Deutsche Bahn particularly explored the AI method known as Deep Reinforcement Learning, in a research project funded by the German Ministry for the Environment. The title of the project “KI am Zug” is a pun noting that the time for using AI has arrived, especially in the context of trains. The results from the project are a crucial step towards an automated Capacity & Traffic Management System (CTMS), which—combined with other components of Digital Rail—is the basis for more capacity, punctuality, and efficiency in railway traffic.
The challenge for railways in the coming years will be to optimally plan substantially more traffic without building new tracks. When thousands of trains are driving in dense traffic, quick decisions on how to adjust operations need to be made as disruptions happen. Those decisions can lead to a cascade of further operational adjustments, as the safe movements of trains on a shared infrastructure are highly interrelated. This level of complexity is driving the current mostly human-operated planning and dispatching processes to their limits. So far, these existing processes have only been supported by IT to some degree, due to the limitations set by the lack of scalability of conventional methods. Optimization tools that work for small portions of the network, and have a local focus, cannot be applied to larger networks, let alone the entire German railway system—a system with several ten thousand train rides per day on more than 33.000 km of tracks—without incurring unacceptably long computation times.
According to the results of “KI am Zug”, the investigated approach of Deep Reinforcement Learning fulfills this requirement of scalability. Current AI optimizers are able to process requests at scales of at least 300 trains running on railway corridors with over 3000 kilometers of tracks within a very short time. The optimizers generate highly detailed operational plans, providing information at the microscopic level for train movements and for the infrastructure control and safety system. The CTMS prototypes are capable of quickly reacting to disruptions, currently simulated, by re-planning the original operational plan. This means changing the trains' routes, changing the order in which trains enter a switch, or adjusting their speed profiles, as needed to restore a smooth flow of traffic. The required computation time grows only proportionally with the problem size and not exponentially. This is a strong indicator for the desired improvement in scalability over classical optimization methods.
Scalability is made possible by the reduction of complexity in learning AI systems. In Deep Reinforcement Learning, the AI system learns by interacting with a simulated railway environment and improving its ability to make sound decisions in a given situation, as the system’s experience grows. For each situation, the AI system is provided detailed track configurations as well as the physical movements and service intentions of trains on these tracks. This training process exposes the AI system to a large variety of situations, and the simulated environment evaluates the quality of the AI system’s decisions in terms of desired indicators such as travel times, travel distances, punctuality, and compliance with various constraints. In this way, many different types of optimization contexts and border conditions can be modelled and trained. Such modelling flexibility is another fundamental feature of Deep Reinforcement Learning, in addition to the previously mentioned scalability. Furthermore, from the “KI am Zug” project, these AI systems have proven the feature of generalization. Extensive experiments with artificially generated track networks have shown that trained AI systems successfully apply the skills from their training process to new tasks that were not experienced in the training phase.
In addition to planning and controlling how trains run on the railway infrastructure, some of the CTMS prototypes developed already integrate the scheduling of track maintenance. When a CTMS optimizes traffic and repair at the same time, both needs can be served better, as was demonstrated by experiments in “KI am Zug”. This CTMS function is primarily being developed for long-term railway planning, where construction schedules are made years in advance, with the objective to minimize the impact of construction on capacity and service.
Applying Deep Reinforcement Learning is still pioneering work, as there are hardly any examples of it integrated within current industrial production environments. By developing the CTMS for the infrastructure management and conducting projects with train operators, such as “AI in S-Bahn systems”, Deutsche Bahn is breaking new ground. Complicating matters even more, this type of artificial intelligence approach required a realistic virtual learning environment. For this purpose, the CTMS team of Digitale Schiene Deutschland developed a microscopic high-performance simulation of railway operations, which was further tailored to the specific requirements of AI training and quality assurance by “KI am Zug”. Both this simulation environment and the AI systems described continue to be under active development with steady progress towards the eventual target of managing capacity and traffic at the scale of the entire German railway network. Building off of the fundamental results in scalability, flexibility, and generalization, as demonstrated in the project, the Deep Reinforcement Learning approach promises to be a game changer for the future railway system.